“Find the set of portfolio weights that maximizes risk-adjusted return.” Over the decades, this foundational framework has guided countless investment strategies, particularly those that deviate from traditional market-cap-weighted approaches.
Markowitz’s solution required three key inputs:
- Predicted stock returns,
- Predicted portfolio risk, and
- Constraints to manage diversification, concentration, and turnover.
While methods for predicting portfolio risk have advanced significantly—thanks to stable correlations and robust factor models—return prediction has proven far more elusive. Traditional linear approaches, such as the Capital Asset Pricing Model (CAPM) or fundamental factor models, often fail to produce reliable out-of-sample results. This gap in return prediction has limited the effectiveness of portfolio optimization for decades.
The NxtGen Index Series: A Modern Approach
Enter the FT Wilshire NxtGen Index Series, which harnesses recent advances in machine learning (ML) to address this challenge. By applying sophisticated statistical techniques, NxtGen delivers more robust return estimates, transforming portfolio optimization into a more effective tool for modern investors. The series draws on three cutting-edge MLmodels:
- Linear Ridge Regression, which minimizes overfitting by penalizing complex solutions.
- Gradient Boosting, capturing non-linear relationships through sequential decision trees.
- Random Forest, an ensemble method that balances bias and variance for consistent performance.
What sets these models apart is their ability to navigate high-dimensional data, adjust for overfitting, and uncover non-linear relationships—factors that traditional models struggle to address. By incorporating 105 financial metrics across 12 categories, including Value, Momentum, Liquidity, Productivity and Default Risk, the NxtGen Index Series offers a more comprehensive approach to solving Markowitz’s original optimization problem.
Real-Time Adaptability and Broader Insights
Beyond predictive accuracy, the NxtGenIndex Series stands out for its adaptability. Factor exposures are recalibrated dynamically, ensuring relevance across economic cycles. For instance, as underperforming factors like Size lose importance, exposure shifts toward improving factors such as Value.
Additionally, the series is trained on a global dataset, enabling it to capture nuanced patterns that regional models might overlook. This broader perspective strengthens the framework’s ability to generate robust estimates, improving overall portfolio construction outcomes.
The Real-World Impact
While traditional models have struggled to balance risk and return, empirical results from the NxtGen Index Series highlight its effectiveness. By delivering more reliable return estimates, the indexes have consistently shown superior performance compared to market-cap-weighted benchmarks, alongside lower volatility and improved drawdowns.
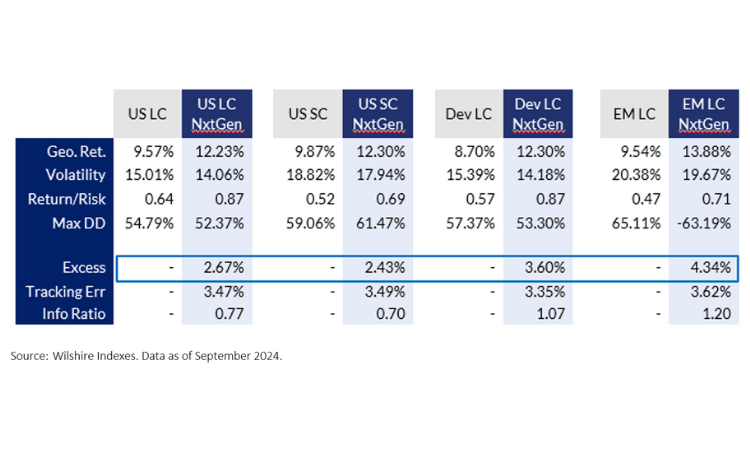
For a deeper understanding of the methodologies and their applications, here is our recently published paper that delves into the innovations driving this series. It explores how machine learning has redefined the return estimation problem and provides detailed insights into the methodology.
A Step Forward in Portfolio Optimization
The FT Wilshire NxtGen Index Series demonstrates how machine learning can solve one of portfolio optimization’s most persistent challenges. By addressing the return prediction problem with modern tools, it delivers a sophisticated solution for investors navigating today’s complex markets.
Learn more about the FT Wilshire NxtGen Index Series at: https://www.wilshireindexes.com/index-research-papers/leveraging-machine-learning-in-portfolio-construction